Discovering Drugs with the Help of Machine Learning
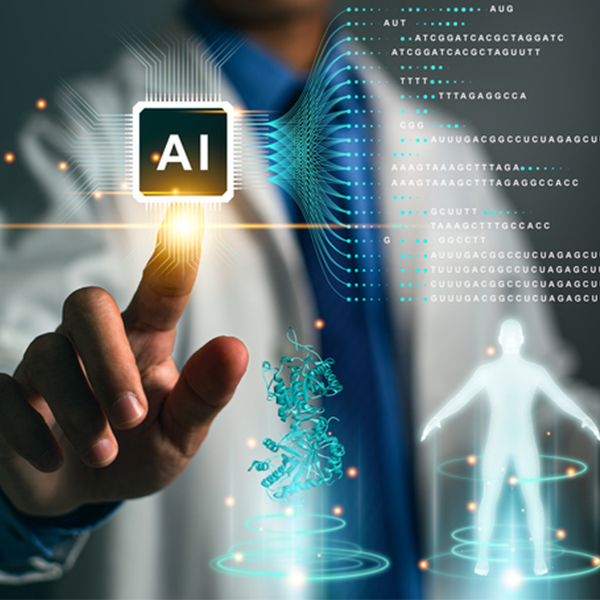
Person in white coat pointing to AI button (Suriphon Singha, Getty Images)
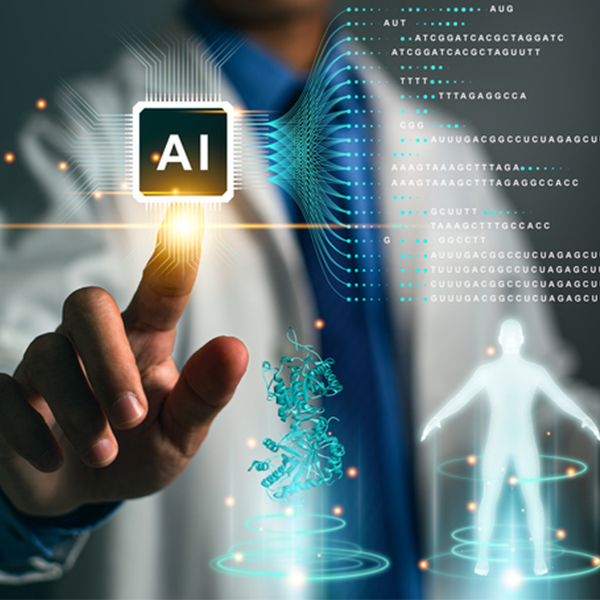
Person in white coat pointing to AI button (Suriphon Singha, Getty Images)
6.6
How does this align with my curriculum?
BC
12
Anatomy & Physiology 12 (June 2018)
Big Idea: Gene expression, through protein synthesis, is an interaction between genes and the environment.
YT
12
Anatomy & Physiology 12 (British Columbia, June 2018)
Big Idea: Gene expression, through protein synthesis, is an interaction between genes and the environment.
BC
12
Anatomy & Physiology 12 (June 2018)
Big Idea: Homeostasis is maintained through physiological processes.