Bias and Sources of Error
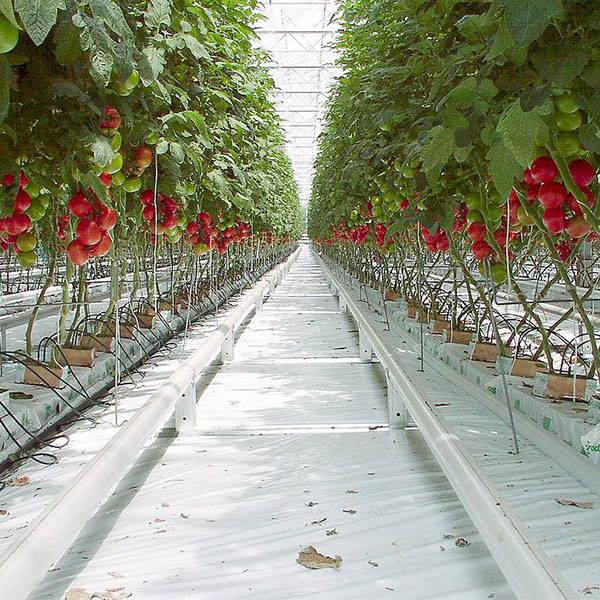
Tomato plants grow in a greenhouse (Goldlocki, Wikimedia Commons)
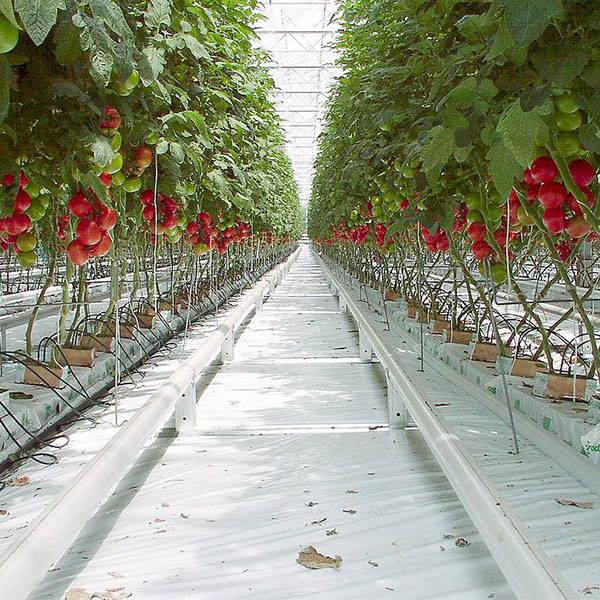
Tomato plants grow in a greenhouse (Goldlocki, Wikimedia Commons)
How does this align with my curriculum?
Grade | Course | Topic |
---|
Learn how scientists identify and minimize bias and sources of error to produce accurate results.
Bias and Sources of Error
In a Fair Test, it is important to work very hard not only to control the variables, but also to minimize sources of the experimenter’s bias and error. Bias and errors can result in inaccurate results from an experimental inquiry.
How can bias occur in experimental inquiries?
In an inquiry, bias occurs when a person influences the results, which in most cases is not intentional. People generally find what they expect to find, because they look for specific things and inadvertently overlook other things, which leads to biased results. This type of bias happens to us all and it is very difficult to control – even for scientists! The background knowledge and prior learning we bring to the situation will affect how we interpret the information we receive. Students in particular may want their hypotheses to be proven to be true by their observations and so may unintentionally introduce biases into their experimental inquiries.
Randomization
One type of bias involves giving preference to a certain group or part of the data. For example, students may hypothesize that one treatment (e.g., giving plants more water) will make plants grow taller than another treatment (e.g., giving plants less water). When students then choose seeds to grow for this experiment, they may unconsciously choose the largest seeds for the treatment that will receive more water and the smallest seeds for the treatment that will receive less water. In this case, the students may be unconsciously trying to give the seeds a head start by choosing the larger seeds.
How to avoid this type of bias:
Have the students randomly choose seeds used in the treatments. Through the process of randomization, objects or individuals are randomly assigned to experimental groups. In this way, the experimenter does not show preference (or bias) to any one group.
Blind Experiments
Sometimes other subconscious biases can occur during experimental inquiries. Some of this bias can be minimized using what is known as a Blind Experiment or Blind test. Blind experiments are often used when an object or group generates some sort of feeling in the experimenter. For example, on the Fair Test page there was reference to two types of seeds called “Big Beauties” and “Small Wonders.” Given these names, students may not look after the plants in the same way. They may try to look after the “Big Beauties” more carefully or give them some sort of unfair advantage.
How to avoid this type of bias:
Have objects or individuals used in experiments labelled by letter (e.g., A, B) or number (e.g, 1, 2) rather than by name, in the same manner as the seeds used in the TomatosphereTM Seed Investigation. In this way, the experimenters (students) will not know which group each member belongs to and will be more likely to treat groups in the same way.
How can errors occur in experimental inquiries?
No one is perfect! That is why errors can happen even in the most carefully designed experimental inquiries. Knowing the types of errors that are common in experimental inquiries can help students to minimize them.
Mistakes
These types of errors are also known as “blunders” or “miscalculations” and they happen to everyone. In experimental inquires, these types of errors can occur due to:
- incorrect reading of instructions (e.g., 50 mL vs. 500 mL, sugar vs. salt, etc.);
- incorrect measuring (e.g., inches instead of cm, °F instead of °C, voltage instead of current, etc.);
- incorrect reading or use of instruments (e.g., meniscus, calipers, thermometer, etc.);
- incorrect calculations (e.g., dividing instead of multiplying, using the wrong formula, etc.); and
- incorrect recording (e.g., transposing numbers, putting values in the wrong place on a chart, etc.).
How to avoid these types of errors:
Encourage students to be careful when they read instructions, take measurements from instruments such as rulers or thermometers, perform calculations, and record observations. When working in groups, encourage students to check and confirm each other’s measurements and calculations. They should also practice taking readings or using equipment prior to doing so in an experimental situation.
Other types of mistakes are possible as well. They include such things as:
- accidents (e.g., knocking over a plant pot and having to scoop the contents back in, giving a plant too much water, etc.); and
- not following directions (e.g., watering some plants every day and other plants every other day, forgetting to water some of the plants, etc.).
How to avoid these types of errors:
Encourage students to undertake experimental inquiries carefully and to the best of their abilities. Having a clearly defined plan and well laid out work area for inquiries will help minimize accidents.
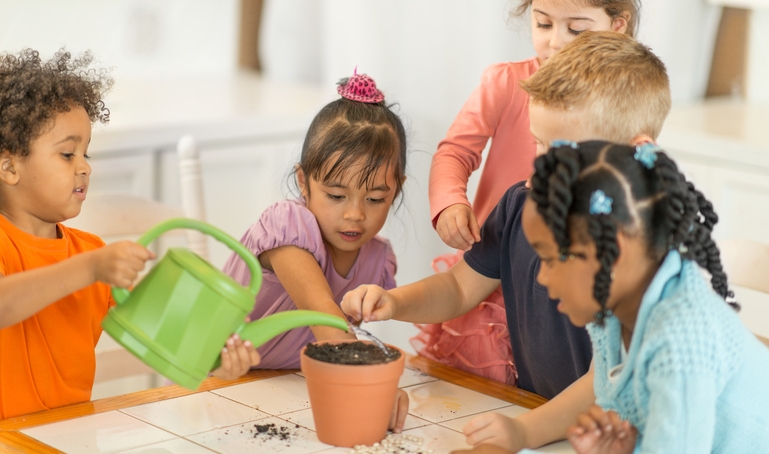
Image - Text Version
Shown is a colour photograph of a group of five young children, about five years of age, helping to water a plant.
A clay plant pot filled with soil is in the centre of a square table. On the left, a child with curly brown hair and an orange t-shirt holds a green watering can above the pot with both hands. In the centre, a child with dark brown hair in a pink barrette, picks up seeds from the table around the pot. Further right, a child in a pink frilly dress reaches towards the pot. Next, a child with short blond hair and a black t-shirt is placing a seed in the soil. On the far right, a child in a blue sweater with black hair in several thick braids, looks at the pot with curiosity.
Experimental Errors
In addition to mistakes, there are other types of non-human errors that are related to the accuracy and precision of measurements. These are known as Experimental Errors. Experimental error is the difference between a measurement and its accepted value. There are two main types of experimental error – Systematic Error and Random Error.
Systematic Errors
Systematic Errors affect the accuracy of a measurement (for more, see the Precision and Accuracy backgrounder). Errors of this type tend to result in measurements that are consistently too high or consistently too low. For example, a digital scale that reads 102 grams for a 100 g standard weight, a clock that is running slow, or a thermometer that has a crack in it.
How to avoid these types of errors:
Systematic errors can be difficult to detect, but the more students understand how to use and calibrate given tools and take observations effectively, the more these types of errors can be reduced. Also, encourage students to use a “gut check” – e.g., “this scale reads 102 g and I know that the weight should be 100g, so the scale needs to be calibrated.”
Random Errors
Random Errors affect the precision of a measurement (for more, see the Precision and Accuracy section). Unlike systematic errors which tend to give results that are always either too high or too low, random errors can give results that are sometimes too high and sometimes too low.
For example, a student is using an ammeter to measure current in a given circuit. The results the student gets are 0.16 A, 0.15 A, 0.17 A, and 0.14 A. Clearly, there is a wide range of results, which should not be the case if no variables were changed when the readings were taken (i.e., mathematically, the reading should have been 0.15 A). The causes of random errors can be difficult to predict and it can take time to figure out the source of the errors. Random errors tend to be common when studying living things as they can be individually affected by changes to the environment; such as variation in temperature, humidity, light, etc. and there is natural variation as a result of their genetic makeup.
Random errors, also known as random variation, are an intrinsic part of any measurement. For example, the ammeter readings above have a percent average deviation of 6.7% (which, for ammeters, isn’t all that bad) so the final reading could be reported as .15 A ± .01 A (6.7% of .15).
How to avoid these types of errors:
Random errors can be minimized by checking to make sure equipment, such as electrical measuring devices, are in good working order. It can also be minimized by using materials from the same source (e.g., seeds from the same package), collecting data at the same time of day, etc.
How are Bias and Sources of Error Minimized in Tomatosphere™?
The TomatosphereTM Seed Investigation has been designed to minimize some of the types of bias and errors described above.
Did you know?
The Tomatosphere™ Seed Investigation is a Single-blind experiment. This means that the researchers at Tomatosphere™ know which seeds are which, but that the classes participating in the inquiry do not (until they have submitted results).
The Seed Investigation is also a Blind Test. In any given year, educators and students will not know which seeds have been into space (or treated with space-like conditions) and which have not since they received two packages of seeds labelled with different letters. Once educators submit their results to Tomatosphere™, they find out which seeds are which. This should avoid any bias students may have towards the ‘space’ or ‘non-space’ plants.
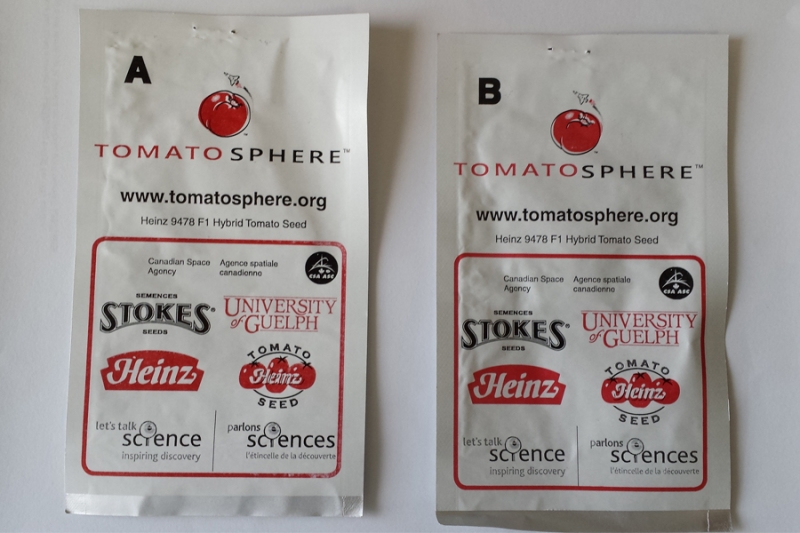
Image - Text Version
Shown is a colour photograph of two packets of Tomatosphere seeds.
The packets are white with red and black printing. They are identical except for a bold letter A printed in the top left corner of the one on the left, and the letter B printed in the top left corner of the one on the right. At the top of each is an illustration of a red tomato with a space shuttle flying around it. The word Tomatosphere is below in block letters. www.tomatosphere.org is printed below that. At the bottom is a red outline of a box with six logos: Canadian Space Agency, Stokes Seeds, University of Guelph, Heinz, Heinz Tomato Seed and Let's Talk Science/Parlons Sciences.
Also, within a class and the Seed Investigation as a whole, many samples are collected. This large sample size helps to minimize random errors generated by the small environmental differences to which the seeds are subjected.
For more information, see the Sample Size and Reproducibility backgrounder.
Guided Practice
Exercise 1
Have students read the following examples and identify which type of error is occurring as well as what could be done to prevent the error.
- A balance scale reads 0.25 g even when there is no mass on it, but students use the scale the way it is.
- The student who is assigned to watering the Tomatosphere™ plants forgets to do so for one week.
- A student uses a measuring spoon to carry water to the plants (but sometimes the water spills a bit…)
- Students measure the dry mass of three 8 week old Tomatosphere™ plants and get measurements of 131 g, 136 g, 127 g.
Exercise 2
In their own words, have students explain the difference between “bias” and “error” as well as between “systematic errors” and “random errors”.
Exercise 3
Have students think about and record the errors that they know, or believe, to have occurred during an experimental inquiry in a log such as a science journal.
Exercise 4
When students plan inquiries, have them list potential errors and sources of bias that might occur as well as ways they could prevent them as part of their method.
Answers
- Type of error: Systematic error
How to avoid: Calibrate the scale. The scale should read 0 when there is no mass on it, and it should measure a standard mass correctly - Type of error: Mistake (not following directions)
How to avoid: Clearly outline tasks, such as watering, to students. Have students check a chart when they have completed tasks.
How would you deal with this situation (forgetting to water for one week)?
If only one plant had not been watered, then students could use data from the other plants. If all the plants were not watered, the experiment should be done over again. - Type of error: Mistake (accident)
How to avoid: Move the water source closer to the plants or use a different type of measuring device that is less likely to spill when carried. The accessibility and location of materials can be optimized to reduce accidents. - Type of error: Random error
How to avoid: Measure the dry mass of more plants if possible. Variations in dry mass are affected by how well the water is removed from the plants before taking measurements.
Learn More
Bias
Research Bias
This article by Explorable explains design bias, selection/sampling bias, procedural bias, measurement bias, interviewer bias, response bias, and reporting bias.
Avoiding Bias
This page by 3Rs-Reduction.co.uk explores multiple ways to avoid statistical bias.
Experimental Design
This page from Yale University explains the different elements of experimentation.
Errors
Experimental Errors and Uncertainty
This PDF from the University of Rochester explains how to use refined experimental methods to avoid errors in measurement.
Random Errors
This entry in AQA Science Glossary defines random errors using the example of Aaron’s Table.
Systematic Errors
This entry in AQA Science Glossary defines systematic errors using the example of a wrongly calibrated instrument.
Minimizing Systematic Error
This page from Cornell University explores different ways to avoid error in experimentation.
Identifying Potential Reasons for Inconsistent Experiment Results
This video (5:03 min.) by Study.com explains how planning for error and inconsistent conditions can minimize inconsistent results.